Medical imaging covers a wide range of algorithms and applications focusing on the processing of medical imaging data. Medical imaging data range from 2D images, such as X-rays and ultrasound images, to multidimensional volumetric data (3D/4D) from various modalities, such as Computed Tomography (CT), Magnetic Resonance Imaging (MRI), Positron Emission Tomography (PET), Single-Photon Emission Computed Tomography (SPECT) and functional MRI (fMRI).
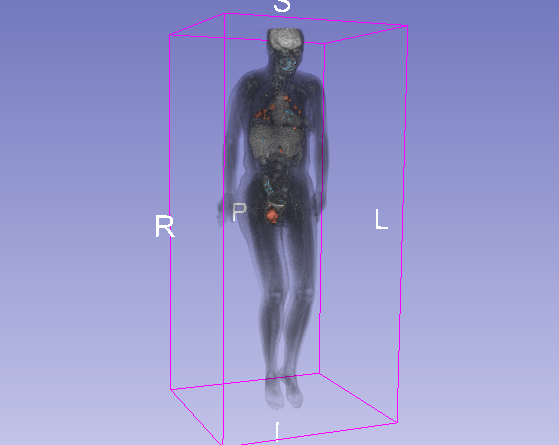
The most common applications of medical image processing include contrast enhancement or correction (histogram equalization, contract matching), image segmentation (threshold-based, region growing, watershed, Markov Random Fields and similar classification-based methods), image registration (rigid or elastic models, based on the intensity of the aligned images or on the geometrical properties of selected key points), digital subtraction radiography (for highlighting the differences between two or more compared medical imaged or data sets).
Medical imaging algorithms are also applied as pre-processing steps to more complex applications, such as Machine Learning-based classification (feature detection and selection) or medical data visualization.
Identifying and delineating regions of interest within an image, such as tumor boundaries or organ structures for treatment planning and diagnosis. Monitoring disease or treatments progression through the longitudinal analysis of 3D images, e.g. PET/CT analysis for Metastatic Melanoma progression assessment.
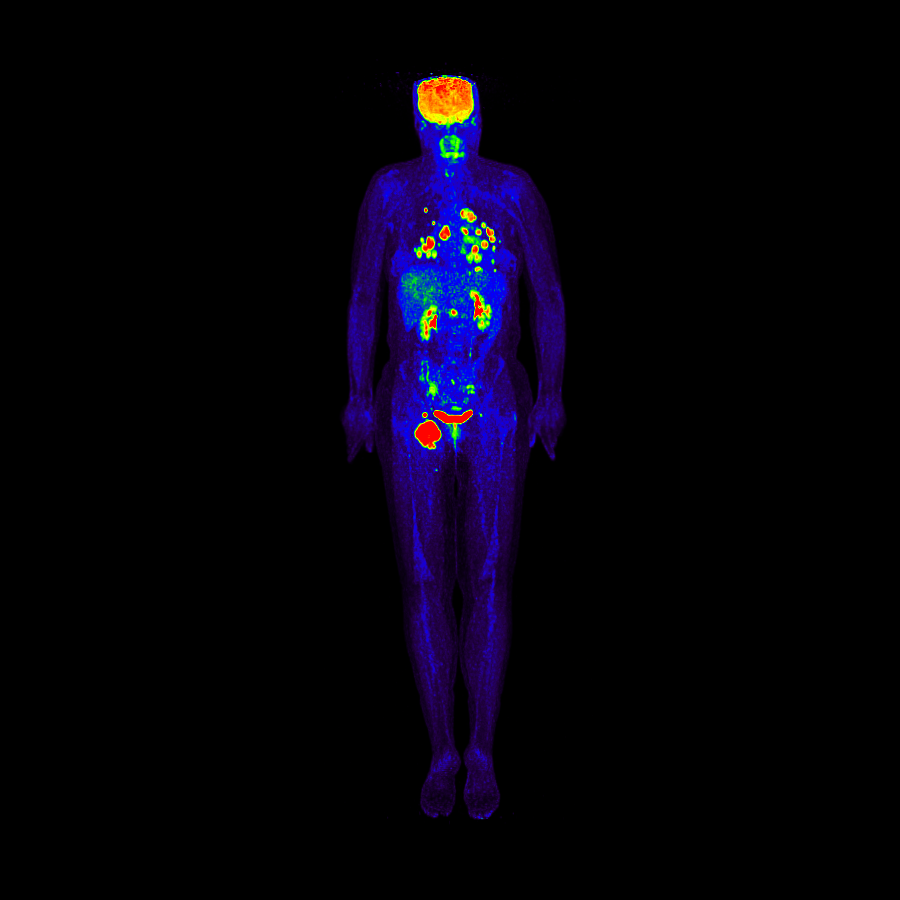
Scanner Parameters: fMRI: voxel-size 2x2x2mm3, 60 slices, FoV 204x204x120mm3, TE=50ms, TR=1.7s, FA=84o, Multi-Slice factor 4
Also acquired: T1 MPRAGE, T2 dark fluid, field maps
Analysis Parameters: discard 3 first volumes, motion correction, B0 fieldmap correction, brain extraction, spatial smoothing 4mm, highpass filter 50s, ICA to discard noise components, registration to T1 mprage with Boundary Based algorithm. Task box plot was convolved with a Gamma function peaking at 4.5s and used in the GLM as the regressor of interest. Six motion parameters for the movement and the temporal derivative of the task were used as regressors of no interest. The threshold was modified in each task accordingly and the conjunction map was created. The final results include only the activations found in all three tasks.